What Is Retrieval-Augmented Generation (RAG)?
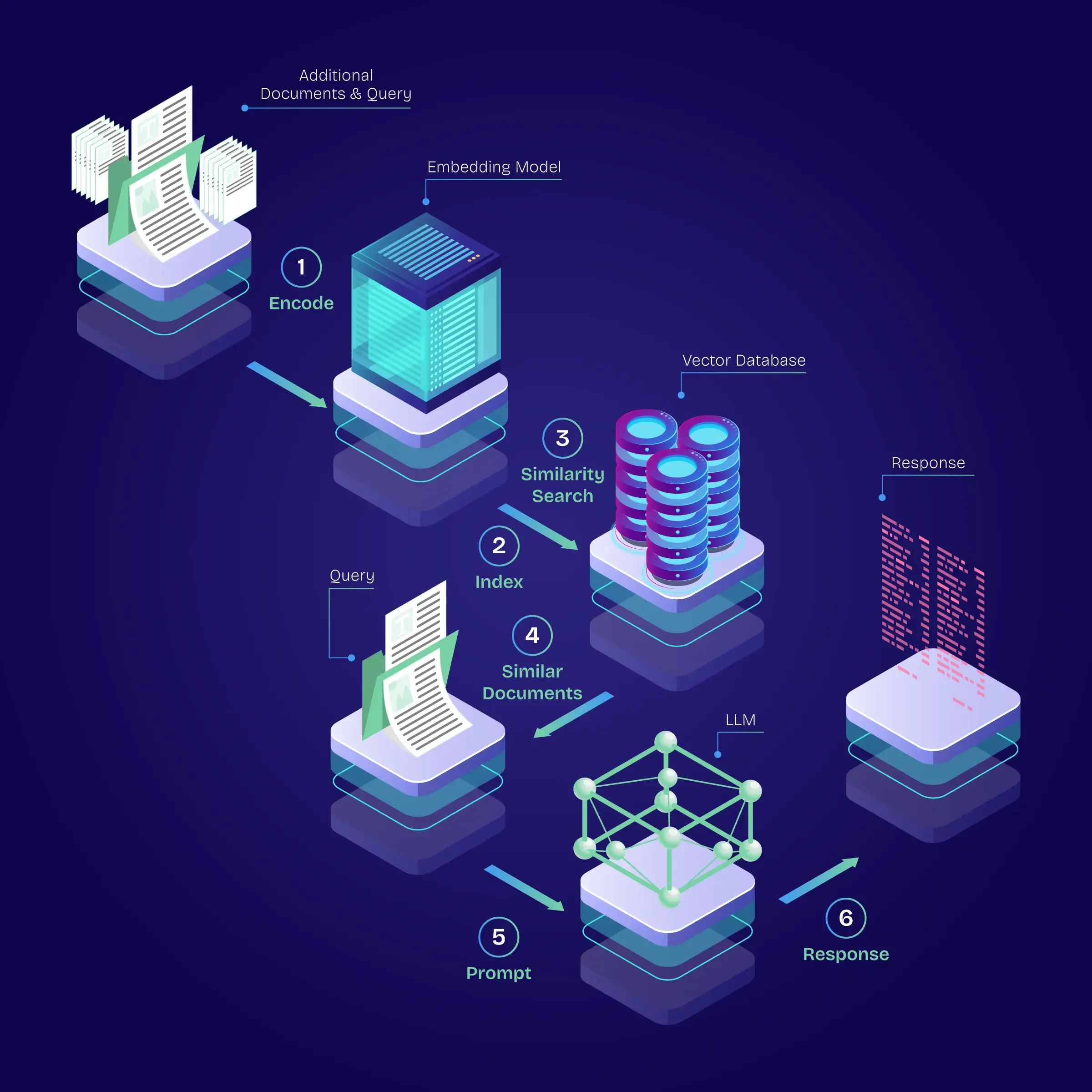
Retrieval-augmented generation, or RAG, essentially means extending and augmenting the capabilities of generative AI by adding a knowledge base. This way, rather than pulling exclusively from fixed training data, the system can also pull relevant and valuable information from this additional knowledge base. The information is then used to craft relevant responses.
As you can see from the diagram above, information stored in existing documents and structures is encoded into the RAG system. Relevant information is then sourced from this external store of data and used to support the LLM as it delivers its generated response. This is still a generative process, but the AI application is feeding off trusted stores of information — the aim is to make the generative AI more useful in an advisory or guidance role.
Why Is RAG so useful in advisory applications?
So what are the actual benefits of RAG, and why is it so useful in delivering advice and guidance? Take a look at a few key advantages.
- Dynamic and up-to-date answers
One of the big problems with static training for generative AI is that there is a cut-off date — a point at which the AI has essentially ended its knowledge. RAG doesn't have this problem. It pulls from current data sources, and there's no training cut-off. A financial advisory chatbot, for example, can work with the latest stock prices and policy changes.
- Domain-specific knowledge without full retraining
Another issue is that using artificial intelligence in new applications often requires significant retraining, which is time-consuming and expensive. General-purpose LLMs, enhanced with additional resources and manuals, make the process much more efficient without sacrificing accuracy.
- Reduced hallucination
Hallucination in AI occurs when the LLM identifies connections, patterns, or structures that do not exist, skewing the output. RAG provides ready-made structures and responses that the LLM can reference during generation. By providing this referenceable framework, RAG significantly reduces the likelihood of hallucination.
You might have noticed a pattern emerging from the above advantages. RAG-supported systems sit somewhere in between pure generative AI and a search engine, forming a hybrid model that hopefully delivers the best of both worlds.
Ensuring accuracy and trust in RAG responses
Now that we know why RAG is so valuable, we need to look at how we can make sure the answers it delivers are accurate and reliable. This requires a considered approach to the enhanced resources.
- Citation features
If you were writing an academic essay, you would need to cite all the sources for your information. An RAG-supported system can be designed to do the same thing. This supports genuine transparency and reliability of the outputs.
- Regular knowledge base updates
If the knowledge base from which the RAG system draws is not up-to-date, the outputs won't be either. Make sure the external knowledge base is regularly updated and ready to deliver relevant outputs.
- Feedback loops
The best RAG-supported systems will feature feedback loops that are built into the system itself. This feature conducts a review of the output before the generation is complete and checks its accuracy. While this can be automated, manual assessment is always valuable too. Human personnel should review outputs regularly and flag any issues that need to be addressed.
In general, ensuring accuracy is actually quite simple. The system needs to be designed to prioritise transparency and accountability, and you need safeguards in place to check that the information and outputs are up-to-date and relevant.
RAG In practice: Real-life examples of retrieval-augmented generation
How can we apply this technology in everyday life and work? Here are a few potential applications.
- Support for human advisors
Perhaps the most obvious application of RAG-supported generative systems is in supporting human customer service assistants in the field. This also provides an additional level of trustworthiness — the system generates the response, and the human operator is able to check it for accuracy and relevance before using it to assist a customer.
Some financial service providers have already begun using RAG in this way. Organisations like Morgan Stanley have said they use the technology to ensure a consistently high level of knowledge and capability right across the company.
- Direct support for customers
If the RAG-supported LLM is trusted, it can interact directly with customers and external stakeholders themselves. A customer support chatbot, for example, is able to deliver responses faster and with greater accuracy if it is backed up with RAG. Whenever new products and services are launched, the external knowledge repository can be updated, with no need for a whole new process of training.
However, automatic and manual monitoring is vital here, as incorrect information delivery is likely to be harmful to your organisation if left unchecked.
- Simplifying high-stakes Information
Healthcare and medical advice and legal guidance are classed as high-stakes information. This information tends to be complex, and there is a high consequence if things go wrong. A well-designed RAG system can deliver information that is easy to understand without sacrificing any accuracy.
As this information is so high-stakes, however, it will need to be checked and assessed carefully. It may be best that the information is provided as a prompt to a trained human operator, giving them an idea of how they can offer advice.
Artificial intelligence as a Guide Towards the Truth
RAG-supported systems are an example of how artificial intelligence becomes a guide that can direct users to the truth — rather than a source of truth themselves. These systems draw upon existing sources of data, and then they generate their own responses accordingly. In this sense, they do far more than a search engine can but also far more than a standalone Gen AI tool could.
For instance, searching an existing database can be time-consuming and confusing, and there's no guarantee you'll find what you need. At the same time, relying on a Gen AI tool to deliver extremely technical, extremely accurate answers is not feasible either. By combining the two, it's much easier to achieve up-to-date, relevant outputs.
To discover more about what's possible with RAG-supported systems, reach out to our team. We can help you achieve accurate outputs every time, without fail.