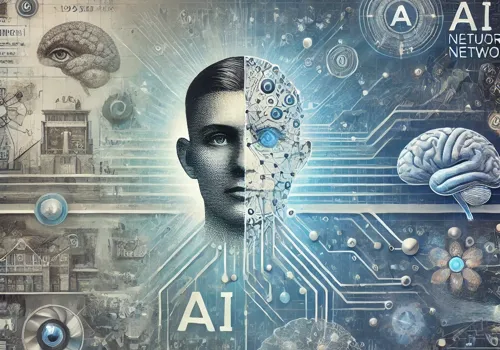
The Evolution of AI
AI is now mainstream and part of our everyday experience at work and home. However, its roots go much further back, starting in the 1950s.
-
- 1950s - The Birth of AI: AI's journey began in this era, marked by Alan Turing's foundational concepts in "Computing Machinery and Intelligence" and the 1956 Dartmouth Conference. It was there that the term artificial intelligencewas first coined.
- 1960s - Early Promise: This decade saw the development of simple AI programs capable of playing checkers, solving geometric proofs, and undertaking early natural language processing tasks.
- 1970s - The First AI Winter: Enthusiasm for AI waned due to the limitations in computational capacity and technical knowledge, leading to a significant reduction in AI research funding and interest.
- 1980s - A Resurgence with Expert Systems: AI gained momentum again by successfully implementing rule-based expert systems across various industries. However, inflated expectations led to a second AI winter towards the end of the decade despite the burgeoning research in neural networks.
- 1990s - The Internet and Big Data Era: The rise of the World Wide Web ushered in an explosion of data, setting the stage for machine learning algorithms like support vector machines to gain prominence.
- 2000s - Mainstreaming of Machine Learning: AI became more integrated into mainstream technology, with notable advancements in speech and image recognition. A significant public milestone was IBM's Deep Blue defeating a world champion in chess.
- 2010s - The Deep Learning Revolution: This period witnessed the advent of deep learning and the use of GPUs, which led to significant breakthroughs in AI applications. CNNs and RNNs emerged as powerful tools for processing complex data patterns.
- 2017 - The Transformer Model: The introduction of the transformer model, as detailed in "Attention Is All You Need," revolutionised AI, particularly in NLP. The rise of large language models (LLMs) demonstrated advanced text generation and comprehension.
- 2020s - The Age of Integration and Ethics: AI's integration into business, healthcare, and everyday technology has become more profound. As a result, there's been an increasing focus on the ethical implications of AI, addressing concerns about bias, privacy, and societal impact.
- The Future: The path ahead points towards advancements in general AI, concentrating on sophisticated natural language understanding and complex problem-solving. Expect ethical, explainable, and human-augmenting AI to be at the forefront of future developments.
The Emergence of Transformers
Transformer neural networks signify a crucial advancement in Generative AI, enabling a more sophisticated and efficient way to process sequential data. This leap forward is mainly attributable to their unique structure, which uses parallel processing, and most importantly, the introduction of attention mechanisms.
The attention mechanisms, a core concept introduced in "All You Need Is Attention," revolutionised how these networks process information. In simpler terms, attention mechanisms allow the network to focus selectively on different parts of the input data, determining which factors are most crucial for the task at hand.
To understand this better, imagine reading a book. Traditional neural networks, like RNNs and CNNs, would read the book sequentially, one word at a time, often losing the context of previous sections. In contrast, a transformer network with attention mechanisms behaves like a reader who can glance at multiple pages simultaneously and understand how words and sentences on different pages relate to each other. This allows for a more comprehensive and contextually aware understanding of the text.
At the initial introduction of the original ChatGPT, it could process up to 8,000 tokens, akin to reading and comprehending approximately 8,000 words or parts of words at a time. The recent development of GPT-4 Turbo, with a 128K token limit, has vastly expanded this capacity. It's like grasping the content of over 300 pages of text at once. While these advancements are significant, transformer models still have limitations in data processing capacity, underscoring the continuous progress and potential for growth in AI.
Today, Transformer neural networks shape the Generative AI landscape. All leading large language models (LLMs) are built upon this architecture, driving unprecedented advancements in natural language processing and understanding.